This is it, boys and girls!
Generative AI is officially done.

It’s a useless piece of tech that outstayed its welcome.
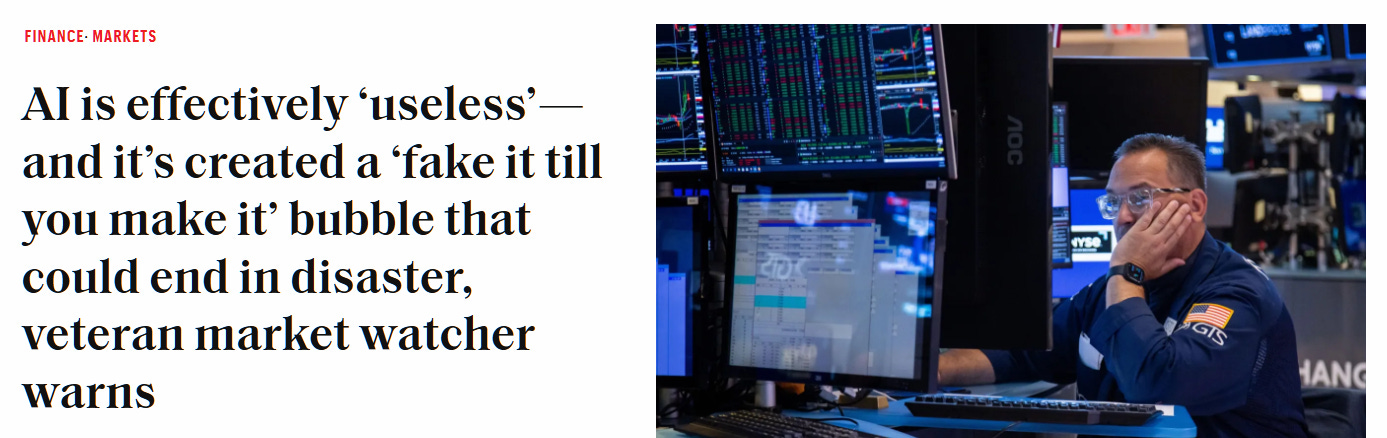
Such headlines are becoming increasingly frequent lately, and I’ll be honest: I get where this sentiment is coming from.
I can sense the vibe around generative AI shifting from excitement to tiredness, doubt, or worse.
Last August, Gartner placed GenAI at the Peak of Inflated Expectations in its Hype Cycle model:
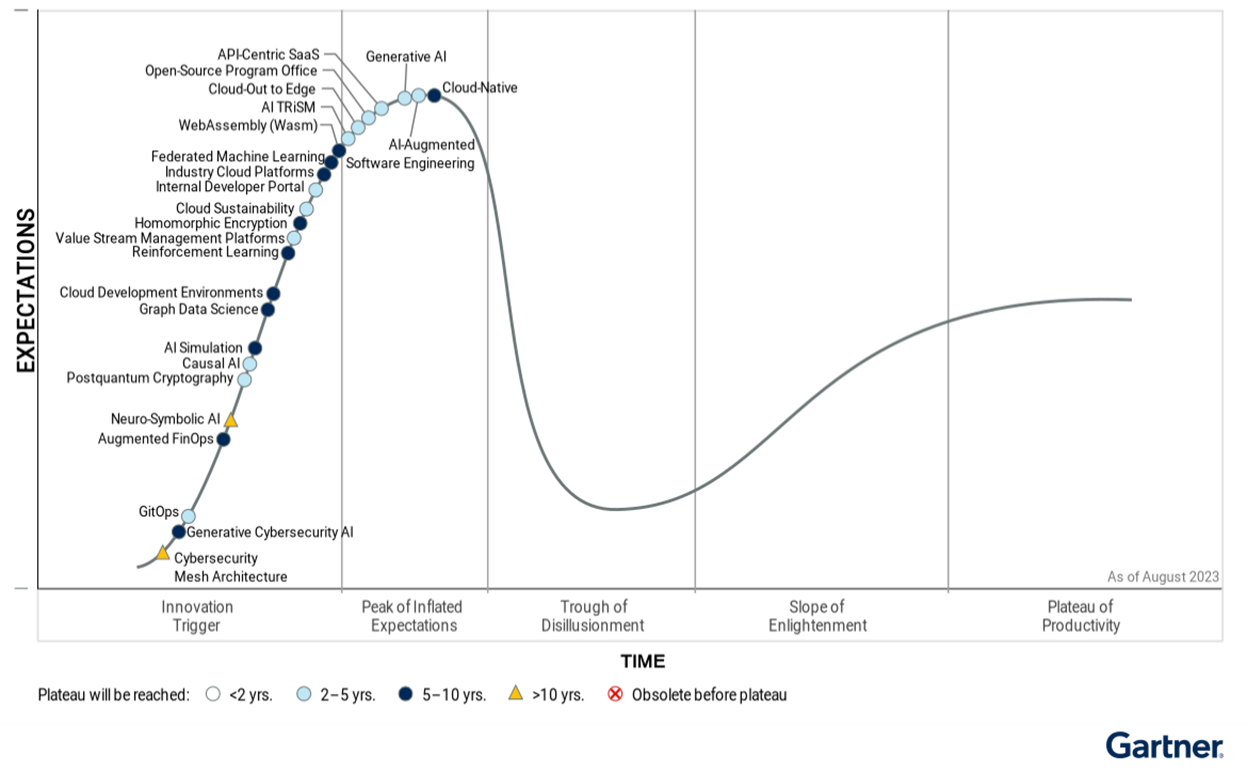
Based on the chatter of recent weeks, I think it’s fair to say we’ve arrived at the Trough of Disillusionment:
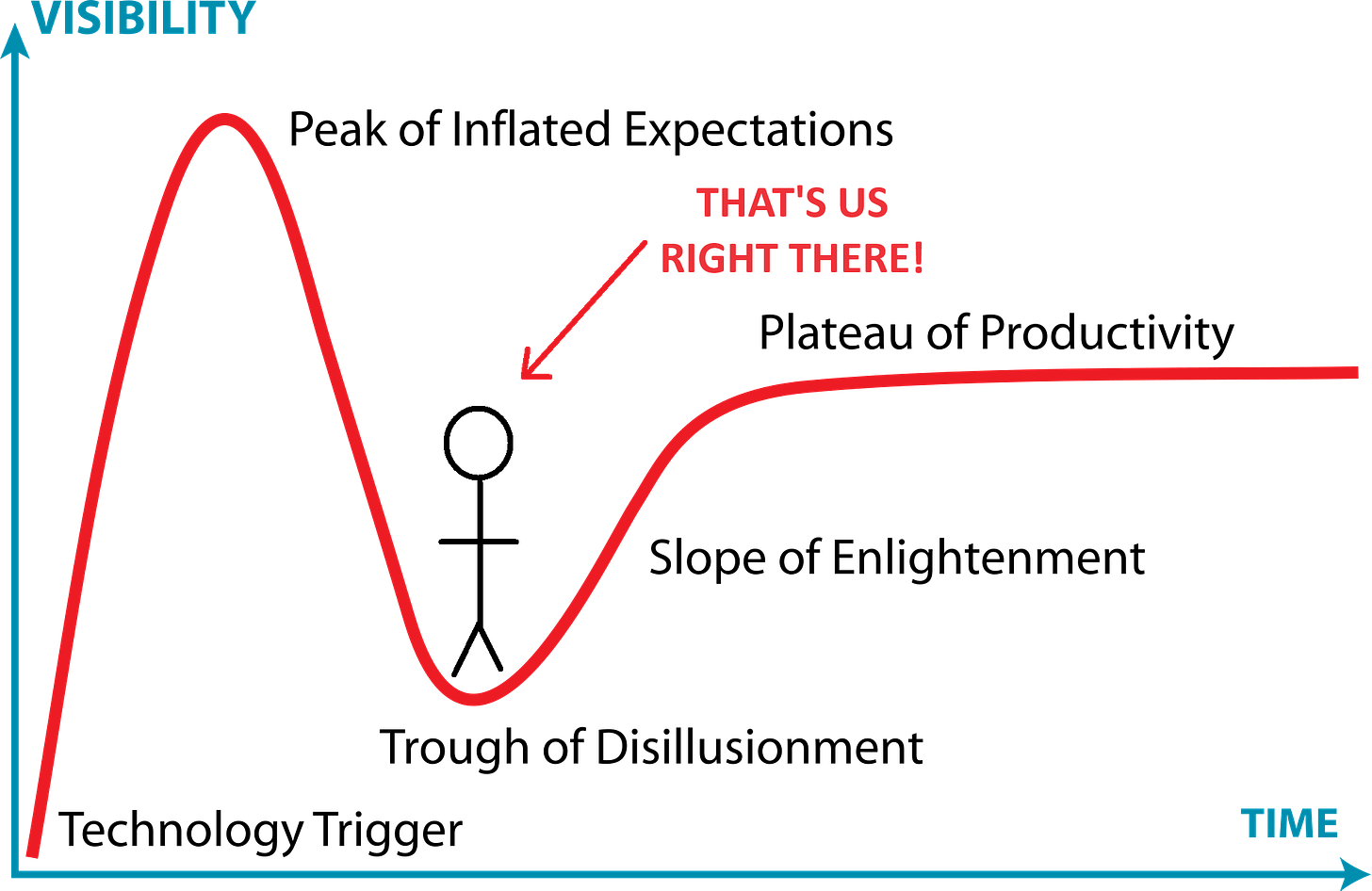
Now, I’m not here to argue that AI isn’t overhyped or that the current bubble won’t burst.
The sheer amount of AI startups that exploded onto the scene during the initial gold rush is unsustainable. Many will go under, dragging their investors with them.
But here’s something I take issue with: All of this doom and gloom creates a persistent impression that generative AI as a whole is a gimmick with no real value.
I think that sucks.
We shouldn’t conflate AI’s inability to live up to unrealistic expectations with its lack of usefulness to the individual.
So I’m here to say: Let’s not throw the proverbial baby out with the bathwater.
Instead, I want us to look to the right-hand side of the Gartner Hype Cycle and set our sights on the Plateau of Productivity, because that’s where we’re eventually headed.1
I’m going to address the sources of the current mood shift.
As far as I can tell, most critical headlines fall into one of three broad categories:
Generative AI doesn’t live up to the insane hype.
Investments in generative AI are not paying off.
The current generation of GenAI—most notably LLMs—won’t get us to AGI.
Let’s break these down.
📈1. GenAI vs. hype
Boy, have we hyped the absolute living shit out of ChatGPT & co.!
I can’t recall any other recent technology that’s been as universally and massively oversold.
I primarily blame the social media hype grifters.
From “Google is done!” claims to breathless “[AI tool] will make [insert profession] obsolete!” threads, you couldn’t even play Candy Crush on the toilet without being bombarded by a dozen hot takes about how AI will change everything and “you’re falling behind.”
It certainly didn’t help that the leaders of major tech companies were all too happy to fuel the flames and feed the narrative. After all, products don’t sell themselves.2
When you put something on this much of a pedestal, it’s just a matter of time before it comes crashing down.
No wonder we’re seeing the mood sour on generative AI and the companies behind it.
Sam Altman—CEO of OpenAI and the poster child of the AI hype train—is currently completing his character arc from unfairly accused “CEO of the year” to “Marvel villain.”
The bottom line is that generative AI never stood a chance of living up to the inflated expectations we’ve collectively manufactured.
Yes, but…
To quote the oft-cited futurist Roy Amara:
We tend to overestimate the effect of a technology in the short run and underestimate the effect in the long run.
There’s little doubt that we’ve overestimated the short-term impact of AI.
But while generative AI can’t match the hype, focusing on that undersells its existing and future usefulness.
Just because a passenger plane can’t fly to Mars, it doesn’t make the fact that it can soar through the air with hundreds of people on board any less impressive.
Just because a smartphone fails repeatedly at helping me hack into a bank vault—uh… hypothetically—it doesn’t take away from how awesome it is at letting me play Candy Crush on the toilet.3
Just because you’re getting tired of these repetitive analogies, it doesn’t mean they didn’t help me make my point.
Silliness aside, generative AI has already proven useful in the real world, from helping fellow Substacker
work on his books to helping other fellow Substackers and do their research to letting yet another fellow Substacker and traditional artist experiment with AI art to changing how this person and this person work with code.So instead of judging GenAI against sky-high expectations, judge the tech on its ability to help you with practical, everyday tasks.
If you’re not quite sure how to go about that, start with the Minimum Viable Prompt:
💰2. GenAI vs. ROI
At the moment, the search for measurable business impacts of generative AI is a mixed bag at best:

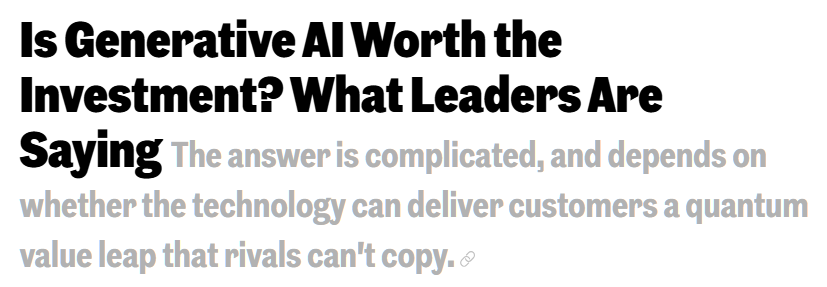
Many AI startups have already crashed and burned. Many more will follow:
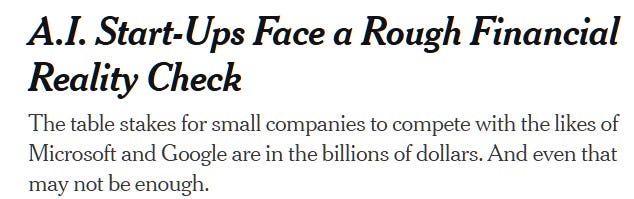
According to Gartner, 30% of generative AI projects won’t make it past 2025.
I’m not the first to say this, but large language models don’t act like typical software:
Their outputs aren’t 100% repeatable and predictable.
They hallucinate and make up plausible “facts,” and even retrieval-augmented generation (RAG) is far from a silver bullet.
Because of this, generative AI doesn’t slot perfectly into business processes that require precision and reliability.
Also, AI doesn’t lend itself well to our standard metrics for measuring the value of business tools.
So yeah, it’s far from a universally rosy picture.
Yes, but…
There are at least two things that existing ROI snapshots can’t tell us:
What the long-term financial impact of generative AI will be.
What “hidden” impact generative AI is already having.
First, we haven’t truly begun to see the business benefits that generative AI has the potential to deliver. It might take years before we do.
But, depending on the source, generative AI is forecasted to bring anywhere from $1 trillion to $4 trillion per year over the next decade.
It’s simply too early to claim that the ROI isn’t there.
As for the second point, a significant portion of AI usage happens outside of any formalized, companywide processes. Microsoft claims that 75% of knowledge workers already use AI but 52% are reluctant to admit they do so.
While this “shadow AI” often entails security risks, it also means companies can’t directly attribute any positive impacts on employee productivity to generative AI.4
As such, short-term ROI estimates might not be representative of the true story.
The bottom line is that we don’t have a clear picture of how much generative AI really contributes to a company’s bottom line.
🤖3. GenAI vs. AGI
The explicit goal of many AI companies is to eventually unlock artificial general intelligence (AGI): the point where AI can perform any task on par with or better than us human meatbags.
And if we’re after AGI, many argue, then today’s large language models are the wrong horse to bet on.
Here’s Yann Lecun, Meta’s Chief AI Scientist:
Cognitive scientist
has been consistently wary of the ongoing AI hype in general and large language models in particular. has pointed out limitations of LLMs that our current LLM benchmarks don’t account for.There’s a growing consensus that we’ll need new approaches to get us to the next level, such as neurosymbolic AI which Marcus is particularly hopeful about.
Yes, but…
Large language models don’t need to be AGI material to be useful.
As I’ve touched upon in point #2, we haven’t even begun to capitalize on the potential of existing models. It’ll likely take a long time to figure out how to make the best of the current generation of generative AI, even if the models never improve.
…we do not need any further advances in AI technology to see years of future disruption. Right now, AI systems are not well-integrated into businesses and organizations, something that will continue to improve even if LLM technology stops developing.
In fact, one can argue that having increasingly capable models that don’t ever reach AGI is in our interest: We’d be getting more competent AI assistants without giving up control or stepping into unknown territory.
That’s the exact case
makes in his excellent “The Goldilocks Zone.”So here’s my take: Don’t ignore the critical headlines. They make valid points.
But don’t let the pessimistic takes stop you from giving AI a try in your own daily life.
So what good is GenAI to you specifically?
In theory, plenty of things.
I covered dozens of use cases in this newsletter over the past two years.
But the truth is, I can’t answer that for you. Only you can.
The best way to learn what GenAI can do for you is to simply give it a fair chance.
One of
’s main principles of using AI at work (and elsewhere) is to “always invite AI to the table.”Do that for a week or so and see whether there are any areas where LLMs, text-to-image models, etc. help you do things faster or better.
Here are some past articles of mine that might give you something to go on, depending on what you’re after:
Images:
Prompting:
Research:
Writing:
Other:
🫵 Over to you…
What’s your take on the current dip in enthusiasm for generative AI? Can you relate to the backlash?
Have you found a way to make AI a part of your daily routine? If so, how, exactly?
Leave a comment or shoot me an email at whytryai@gmail.com.
Gartner’s original graph agrees, giving Generative AI 2 to 5 years to get there.
Unless they’re hot cakes, I am told.
Just kidding, of course. I play Hearthstone.
Ethan Mollick calls such shadow AI users “secret cyborgs” and strongly encourages companies to leverage these employees’ expertise with AI to scale the benefits.
This is getting spooky. Once again my thoughts are just about 100% aligned with yours. I have been saying I am an AI Optimist for a long while now. My optimism is based on real world usage of GenAI tools at work (in cybersecurity) and outside of work.
On the AGI vs Useful AI front, I almost feel like there's a little bit of click bait type headlines approach from at least some writers- while obsessively following and being a very active user in the GenAI space for the last 20-ish months, I have never once expected AGI to arrive imminently. Never once felt impatient to see its arrival; if anything we're often lead to believe that the arrival of AGI will lead to more job losses etc.
For whatever it's worth I'm at the other end of the bored with or disappointed by GenAI spectrum. I am embracing/living this wisdom from Ethan Mollick:
"“always invite AI to the table” is the principle in my book that people tell me had the biggest impact on them. You won’t know what AI can (and can’t) do for you until you try to use it for everything you do. "
To answer the second question, someone who’s better at math than I am should run a regression discontinuity study.